Why you should care about GenAI
June 5th, 2023 by Lorre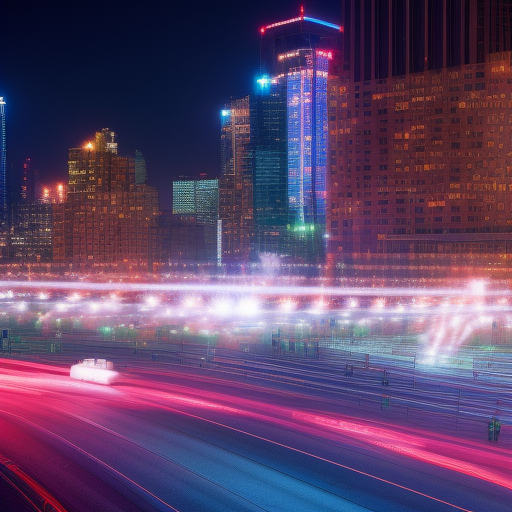
Generative AI (GenAI) empowers end-users to generate content, such as images and text, quickly and easily. Entrepreneurs are taking advantage of this technology to create a growing number of startups that utilize GenAI models for various aspects of content creation. In the coming year, we can expect to see a proliferation of new products that build on GenAI models like titans GPT-3 and Stable Diffusion. The GenAI renaissance is just beginning and the recent boom in niche end-user applications for this technology is just the tip of the iceberg. These models will serve as the foundation for many future applications ushering in a new GenAI-economy replete with add-ons to existing software and entirely new offerings for end-users. With GenAI, the possibilities for content creation are endless and entrepreneurs are poised to capitalize on this powerful technology to revolutionize the way we create and consume media.
There are several foundational models across various modalities such as GPT-4 for text and images, Tabnine for code, Whisper for Speech, Meta’s Make a Video for generating videos and DreamFusion for creating 3D models. These models typially have billions of parameters that have been learned from huge volumes of online content. On top of the foundational model layer is the application layer upon which mulititudes of niche products are being built and offered to consumers. Many of these applications are made avaialble through fine-tuning of foundational models to specific tasks.
Why Now?!
AI has been gaining traction/maturing over the past 10 years, particularly gearing up over the past 5 in the Natural Language domain.
Before 2015, we saw very small, task specific models reign supreme where most models could only perform a single task at a time fairly well e.g. classifying an image or predicting delivery time but not sophisticated or expressive enough for general purpose generative tasks e.g. to generate code/essays.
Then in 2017, Google released the Attention is All You Need paper outlining a new NN architecture called Transformers that could generate superior quality language models. Transformers were more parallelizable, requiring less time to train and so enabling Transformers to be customized to specific domains relatively easily. there was a race to scale models to be larger. In essence, Transformers kicked off the race to scale AI models, and surpassed human performance benchmarks in speech & image recognition, reading comprehension and language understanding. However, models are still difficult to run (due to GPU orchestration), not broadly accessible (closed beta only or unavailable) and expensive to use a cloud service.
From 2020-2022, GPUs (compute) got cheaper and new techniques like diffusion models shrunk the cost of training and running inference. The AI research community continued to develop more effective & larger algorithms leading to better(can perform multiple tasks), faster and cheaper models. During this OpenAI release GPT-1 or Generative Pre-Trained Transformer, a type of Tranformer inspired by Google that generated words (or tokens).
Then in 2022, we saw the release of ChatGPT as a result of the confluence of faster, cheaper compute, maturation of AI research and the availability of talent to push the envelope on training large models. And now we’ve seen an explosion of creative applications on top of easy to use/accessible user interface.
Trends
Smaller more personalized AI
Following the rapid scale up of AI in size, we are seeing a higher number of smaller models emerge, leading to more personalized AI. Model files are typically several GBs large, but with the acceleration of infrastructure efficiency & model architecture, we will soon have models small enough to run smoothly on a smartphone. Users will be able to generate images and text, perhaps even run on their own personal data without a GPU. This is a boon for privacy and global access. Some examples include
- infinite personalized radio where original music is auto-generated to suit your mood (think custom lofi to enhance your creative abilities)
- using augmented/virtual reality (3D assets) to enhance shopping experiences (picture picking out furniture from the comfort of your own living room)
- tailored learning with personalized educational lessons for kids that grow in complexity with the child’s learning ability/preferences
ChatGPT Plugins
Some LLMs such as ChatGPT are currently limited in their knowledge retrieval because it is not connected to the internet and was trained on data up to 2021 so cannot access recent information. Normally, we interact with LLMs through a series of prompts - going back and forth until the user gets a satisfactory answer. To address this, OpenAI released tools called plugins designed to help CHatGPT access-to-date information, run computations and leverage 3rd party services. Right now, there are only 11 plugins from companies like stores, and more all through the use of ChatGPT.
Autonomous AI Agents
Addressing LLMs’ struggle to complete complex tasks requiring long term planning without getting sidetracked. LLMs are also not computers with access to working memory like a CPU/GPU and so cannot manage and execute tasks itself without added infrastructure. One way of tackling the limits of LLMs is to use Autonomous AI Agents such as BabyAGI or AutoGPT, which automate the iterative process of feeding prompts into ChatGPT. You can think of it as an AI task manager that generates a sequence of tasks in a loop untila pre-specified goal is reached. In essence, AI agents can start with a complex task that would take humans a week to complete, condense the prompt and identify subtasks for execution. In the near future, we could see personalized AI agents executing actions on our behalf e.g. buying flights to Bora Bora because it knows it’s on your bucket list or act like an always on-hand executive assistant.
More Data Leaks and Prompt Injections
Many companies have outright banned the use GenAI for their employees to prevent sharing of confidential information. We will see more accidentally leadked trade secrets via LLMs like ChatGPT as people learn the risks before improvement. Prompt injections are security risks associated with LLMs, where third parties can force new prompts into a LLM query without the user’s knowledge or permission. Prompt injections can be used to steal data or to deliver malicious responses. Sadly, there are currently no solutions that solve this problem.
Effect of GenAI on the Economy
Just like any revolutionary technology, GenAI will affect the economy as we now know it. Goldman Sachs estimates that as many as 300M jobs could be affected by AI globally. The Insider reports that Legal and finance jobs are especially at risk, while more manual jobs like those in construction will be minimally impacted. However, I don’t believe that AI in and of itself will steal your job. Although, it may be affected by people that become adept at using AI tools to become exponentially more productive. So I encourage everyone to gain AI assisted superpowers for your artistic work, writing or research since access to LLMs is relatively low in cost.
Limits
While AI-powered personalization offers numerous advantages, there are also challenges and considerations to keep in mind before implementing it. Here are a few of the key challenges to consider:
- Data privacy and security: Collecting and analyzing customer data can raise concerns about privacy and data security. In this regard, businesses should ensure that they are observing data privacy regulations and protecting customer data from misuse.
- Implementation costs: Implementing AI-powered personalization can be expensive, particularly for smaller businesses with limited resources. Businesses should consider the costs of technology prior to adopting it.
- Transparency and trust: With high-profile data breaches and privacy scandals, customers are becoming more cautious about how their data is being utilized. To build trust with their customers, businesses need to be transparent about how they are collecting and using their data.